Winning with Data: Goizueta MSBA Students Shine in Travelers University Modeling Competition
Recently, a team of MSBA students from Goizueta showcased their analytical skills in the Travelers University Modeling Competition, an annual challenge hosted by Travelers Insurance. This prestigious competition tasks university students with developing predictive models using real-world business data, testing their ability to solve complex industry problems. Goizueta’s team not only rose to the challenge but stood out for their innovative approach, earning a spot in the semifinals. Here, we chat with the team about their experience, key takeaways, and the strategies that set them apart.
Case
CloverShield Insurance seeks a predictive model to forecast policyholder call frequency, which would optimize call center resource allocation and reduce operational costs.
About the Team
Roffy 25MSBA, Micheli 25MSBA, and Eason 25MSBA are students from the MS in Business Analytics (MSBA) program at Goizuta Business School. Roffy completed his previous degree at Queen’s University and has had internships as a data analyst in many firms. Micheli graduated from Miami University with a finance degree, and previously worked at a security and venture capital firm as an equity analyst. Eason graduated from Zhongnan University with a degree in International Economics. He believes that the use of data is transforming the world.
What inspired you to participate in the Travelers University Modeling competition, and how did you approach the competition?
Roffy: We participated in the Travelers Modeling Competition to explore the vivid world of data science in the insurance industry. Insurance is one of the first industries to have adopted sophisticated statistics and machine learning to add business value to its operations, and we were really eager to learn how the current trends are reflected in the actual day-to-day practice. The competition also provided the opportunity to learn from fellow competitors, many of whom are PhDs and experts with years of industry experience.
For our model, we leveraged the predicting power of a carefully-tuned advanced boosting algorithm and neural network to capture the complex nature of the data. We then built our prediction pipeline with a focus on understanding the distribution of the target variable and tuned the models accordingly.
Could you share some insights into the experience of competing in the challenge and working with your faculty advisors?
Micheli: We found the faculty’s feedback helpful when presenting our approach. They often questioned the reasoning behind our initial model and challenged us to evaluate whether our decisions were justified. Rather than providing direct answers, the professors encouraged us to think critically.
- Biggest Takeaway: We encourage everyone in this program to participate in challenges, competitions and hackathons like this. This is not only to test what we have learned throughout the program but also to get used to dealing with things that might lead to very interesting outcomes and learn how to handle them.
- Something to Share: Lots of distributions came to our mind at first, such as Poisson and Normal. Then we learned about the Tweedie distribution from one of the participant’s presentations, which is supposed to be a special case of exponential dispersion models and is often used as a distribution for generalized linear models.
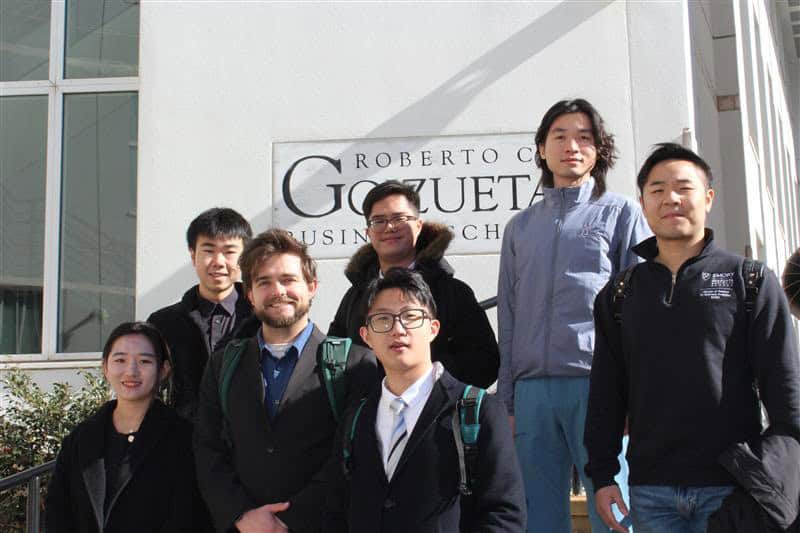
What do you think contributed to your success in advancing to the semifinals? How did you stand out?
Team: Our approach of building a stacked predictive pipeline, which includes a classification model to predict whether a customer will make a phone call and a regression model to predict the exact number of phone calls, was unique among the competitors. This innovation was particularly appealing to the judges.
What advice would you give to future students considering participating in analytics-focused competitions like the Travelers University Modeling Challenge?
Eason: We believe everyone should actively participate in these competitions because, in our future careers, we need to apply data to solve real-world problems rather than simply learning theoretical concepts from textbooks. Competitions like this provide an excellent platform to practice and build a solid foundation for our future work.
How did the coursework you’ve completed in the program contribute to your success in the competition?
Team: We applied all standard model-building methods taught for the competition. Throughout the process, we documented our assumptions, managed variables, and evaluated the model’s robustness. Our coursework in the MSBA program provided guidance on accessing resources and identifying requirements.
Looking ahead, what are your future goals in the field of data analytics, and how do you plan to build on your experience from the Travelers University Modeling Competition to achieve them?
Team: It is a good start to get to know more models that apply to various scenarios. It also reminds us to always keep track of what is advancing in the data analytics field, like new methods.
Interested in exploring MS in Business Analytics opportunities? Learn more about Goizueta’s MS in Business Analytics program.